An interdisciplinary collaboration to decipher the thermodynamic “arrow of time” in complex networks
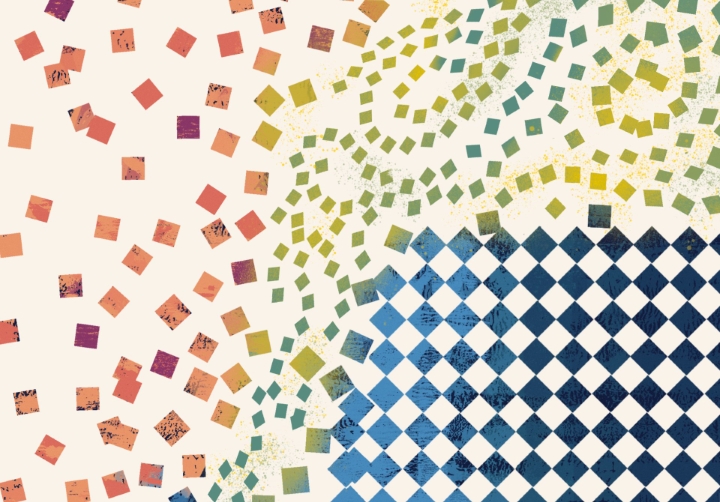
Researchers from the Basque Centre for Applied Mathematics – BCAM, Hokkaido University and Kyoto University have deciphered the thermodynamic “arrow of time” in large-scale complex networks.
This breakthrough is based on the Ising model, a cornerstone of statistical physics and machine learning.
The result sheds light on recent discoveries in biological systems, such as the appearance of an arrow of time in brain signals.
The work has been published in the journal Nature Communications.
New research from the Basque Center for Applied Mathematics – BCAM, Hokkaido University and Kyoto University has found an innovative mathematical solution for the non-equilibrium thermodynamics of large-scale neural networks based on their temporal asymmetry. This breakthrough helps to better understand the behaviour of biological systems such as neural networks, or that of machine learning and AI tools. This research has been carried out by Dr. Miguel Aguilera Ikerbasque Research Fellow at BCAM and Professor Hideaki Shimazaki, associate professor at the Graduate School of Informatics at Kyoto University, and has been published in the research journal Nature Communications.
Life, from the perspective of thermodynamics, is a system in disequilibrium: it continues to maintain an internal harmonic structure while continuously exchanging energy and matter with the environment. According to the second law of thermodynamics, systems tend to move from order to disorder over time. This increase in the level of disorder is known as entropy. If this increase is greater than zero, the system is out of equilibrium and its dynamics has the characteristic of being irreversible over time. This link between entropy and irreversibility was expressed as “the arrow of time” by the English physicist Arthur Eddington in 1927.
The idea of the arrow of time is sometimes exemplified by watching a film in which a crystal glass is broken. As the glass breaks, entropy increases and the system moves to a more disordered state, which looks like a natural sequence of events. In contrast, if we were to watch the film in reverse, it would seem obvious that it is not possible for the pieces to become a whole glass again, going from disorder to order. This seems highly counter-intuitive, since such events require a reversal of the arrow of time, and a decrease in the entropy of the system.
An emerging field of physics, stochastic thermodynamics, has rapidly advanced our understanding of the microscopic non-equilibrium processes that take place, for example, in colloidal particles and molecular motors. These particles and motors are very small systems that play a role in biological systems (e.g. in cell membranes or transporting substances within a cell). However, the non-equilibrium thermodynamics and time arrow of large-scale complex networks, from neural circuits to flocks of birds, remained an enigma… until now.
As a prototypical model of large-scale complex networks, the researchers studied the Ising model, a model of recurrently connected neurons, which is also studied in statistical physics and machine learning. When the connections between neurons are symmetric, the Ising model is in a state of equilibrium and has complex disordered states called spin glasses. The mathematical solution of this state led to the award of the 2021 Nobel Prize in Physics to Dr. Giorgio Parisi. However, unlike living systems, spin crystals are in equilibrium and their dynamics are time-reversible. Instead, the researchers worked on the time-irreversible Ising dynamics caused by asymmetric connections between neurons. The study provides, for the first time, an exact mathematical solution of the temporal asymmetry – also known as entropy production – of non-equilibrium disordered Ising networks.
This research shows that there are two distinct regimes of behaviour that give rise to temporal asymmetry in complex networks. The first is related to the mysterious “edge of chaos”, a critical regime at the boundary between order and disorder. The second is a phase of disorder with quasi-deterministic temporal dynamics. The result bridges the gap between the temporal asymmetry found in the activity of the brain’s neural networks and its underlying mechanisms. “Neuroscientists report that the temporal asymmetry in brain dynamics increases in states of heightened awareness or cognitive effort, but we do not know how it is generated. Our research provides two possible solutions: one based on highly flexible stochastic states that combine order and disorder, and another based on combinations of chaotic but repetitive patterns,” says Dr Miguel Aguilera, Ikerbasque researcher at the Basque Centre for Applied Mathematics.
The exact solutions obtained serve as a reference for developing approximate learning methods for artificial neural networks. The development of learning methods that work robustly in multiple phases discovered in this study will advance machine learning studies. “Ising’s model is a prototype of the artificial neural networks that underpin recent advances in deep learning and generative artificial neural networks. Understanding its behaviour offers critical insights into biological and artificial intelligence in general,” added Prof Hideaki Shimazaki, associate professor at Kyoto University’s Graduate School of Computer Science.
Dr Miguel Aguilera also highlighted the multidisciplinary nature of his study: “Our results are the result of an exciting interdisciplinary collaboration involving physics, neuroscience and mathematical modelling. This unique combination of disciplines has opened the door to new methods for understanding the organisation of large-scale complex networks.
Aguilera, M., Igarashi, M. & Shimazaki, H. Nonequilibrium thermodynamics of the asymmetric Sherrington-Kirkpatrick model. Nat Commun 14, 3685 (2023). https://doi.org/10.1038/s41467-023-39107-y
Link to the article: https://www.nature.com/articles/s41467-023-39107-y