Mercedes-Benz uses Quantum Computing (AYESA) to achieve excellence in vehicle production planning system
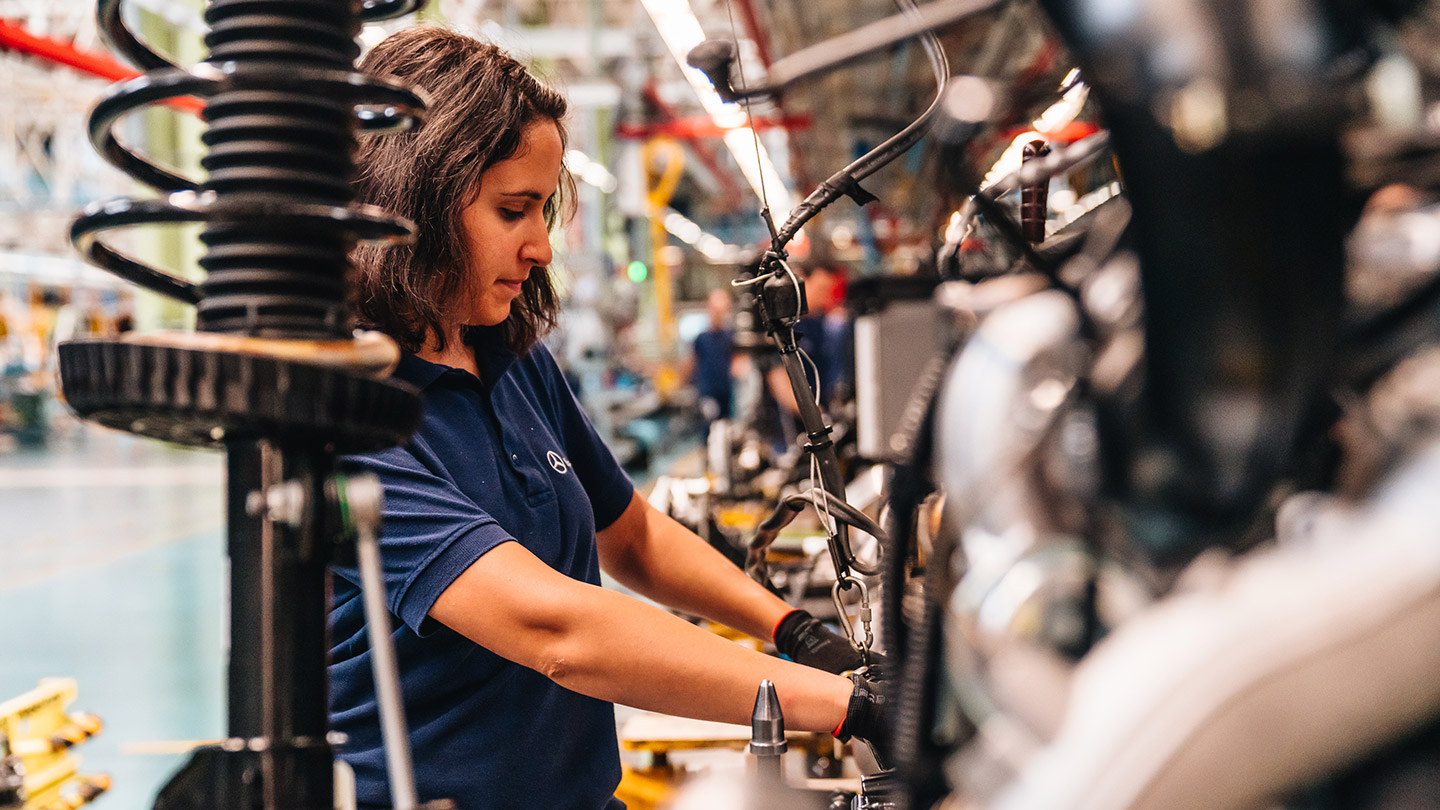
Ayesa develops a platform with classical and quantum Artificial Intelligence to detect any anomaly automatically days before the cars enter the production line.
Ayesa, a global provider of technology and engineering solutions, is working with Mercedes-Benz Spain on a project that aims to achieve zero errors in the car assembly process and achieve excellence in its production system. To this end, it has developed a quantum platform capable of automatically detecting any configuration anomalies several days before the cars enter the production lines.
The Mercedes-Benz commercial vehicle production environment is very distinctive, as each car has an individualised, non-serialised configuration, based on the preferences of each customer. These individualisations enter the system in the form of a list of codes representing the requests made by the customer, whereby each list completely defines a car to be produced.
Until now, the ordering system has tried to prohibit combinations of codes that could cause problems during production, but due to the high degree of complexity due to the number of variables, there may be codes that define a car that cannot be produced. Ayesa’s system identifies cars that cannot be produced, based on these codes, before they enter the production line. The number of false negatives (i.e. undetected cars that cannot be produced) will aim to be zero, also minimising false positives.
Application of quantum computing
The developed quantum model generates an automatic system for the detection of ‘anomalous’ configurations at different hierarchical levels, such as order level, production level, modification level, process level, and the comparison of differences between ‘anomalous states’ and ‘similar states’ at the same date.
The system checks whether the detected difference is correct and consistent with the documentation, and if not, a notification of a possible error is issued, before such an occurrence collapses the production lines in a real plant. In this way, Mercedes-Benz is approaching zero errors in the car assembly process, which means improving the excellence of today’s production systems as much as possible.
In the automotive industry, this is a very complex problem, where the variability of the components that can be combined (more than 5,000 different codes, a large volume of different variants to be manufactured every day), means that it cannot be tackled efficiently and with guarantees using classic IT.
Benefits
Characterising different car profiles according to their modules, as well as detecting, reporting and explaining possible anomalies (strange changes, strange combinations, new code combinations) in preparatory-productive configurations, is one of the most critical, pressing and important challenges in the automotive industry, and currently not effectively solved.
Having an anomaly detection tool helps the review team to filter out the vast majority of vehicles and only check a small number of them. Of all the vehicles checked, some will be anomalous but correct, and others will require some changes to their component list.
In addition, the applicability of anomaly detection in highly complex and highly variable environments, with solutions based on quantum computing and quantum optimisation systems, is extensible to many business areas, such as healthcare and other industries like energy, marketing, distribution, etcetera.
Use case
The proof of concept has been carried out at the Mercedes-Benz manufacturing plant in Vitoria. The system is using a training database, with 50,000 orders (50,000 rows) and each order with 706 different characteristics, i.e. car components, and every day 14,000 orders (test set) are compared with the training set detecting anomalous orders.
To carry out this process, a program has been generated that creates a quantum circuit, based on gate computers, using between 10 and 30 qubits, and has been run on both IBM simulators and real IBM quantum computers, based on the coding of the problem in the Qiskit library.
No previous anomalous orders have been detected in the historical ones, so this is an unsupervised problem, given that new manufacturing is involved. The results of the quantum system are evaluated on a daily basis and the system is constantly evolving. In fact, Ayesa has implemented two models, one for classical AI and one for quantum AI, and is making both compete. Both work well but the quantum one is even more sensitive to anomaly detection.