AI detects cancer and viral infections with nanometre precision
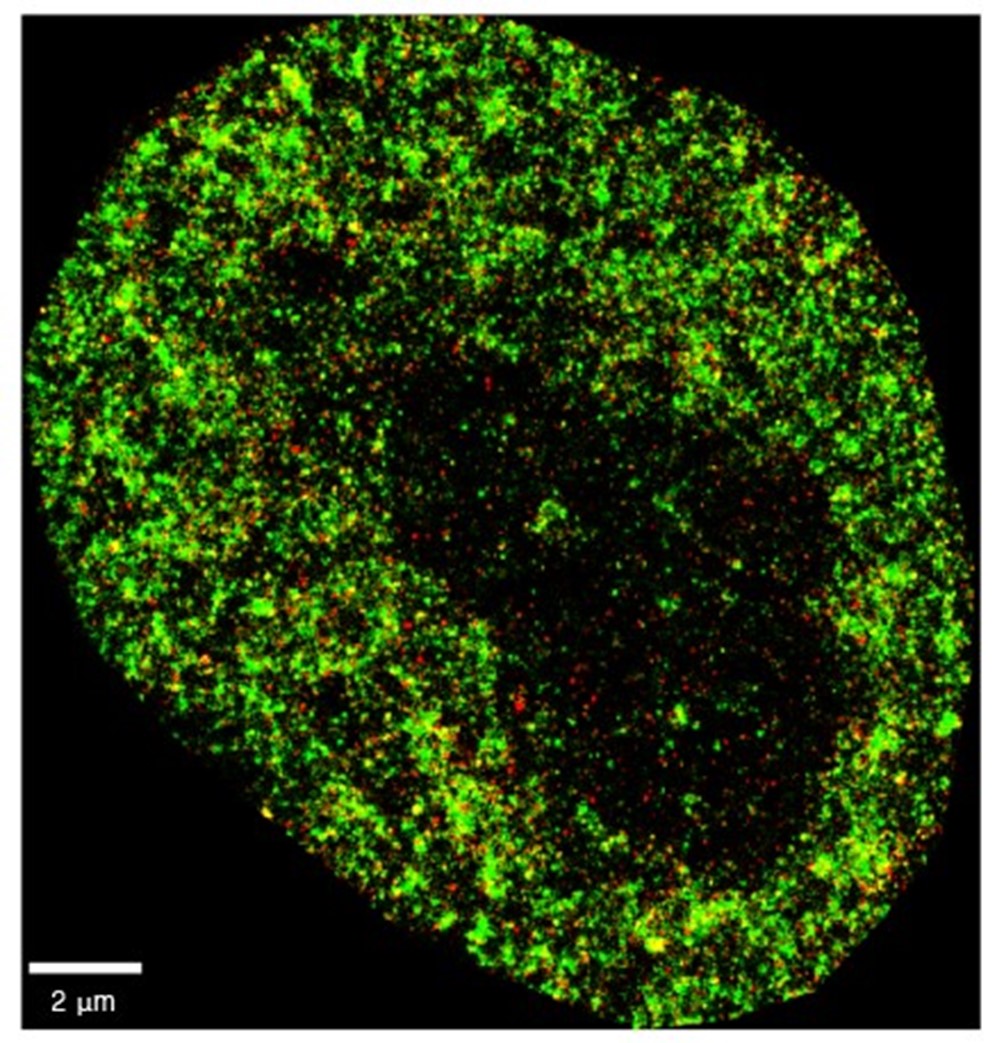
The combination of artificial intelligence with the development of new microscopic technologies to obtain high-resolution images of cells opens the way to new strategies for diagnosing and monitoring diseases.
A scientific team from the Centre for Genomic Regulation (CRG), the University of the Basque Country (UPV/EHU), the Donostia International Physics Center (DIPC) and the Fundación Biofísica Bizkaia (FBB, located at the Biophysics Institute) has developed an artificial intelligence that can differentiate cancer cells from normal cells, as well as detect the earliest stages of viral infection inside cells. The findings, published today in the journal Nature Machine Intelligence, pave the way for the development of new diagnostic techniques and disease monitoring strategies.
The tool, AINU (AI of the NUcleus), scans high-resolution images of cells. The images are obtained with a special microscopy technique called STORM, which creates an image that captures far more detail than normal microscopes can see. The high-definition snapshots reveal structures with nanometre-scale resolution.
A nanometre (nm) is one billionth of a metre, and an individual strand of human hair is about 100,000 nm wide. AI can detect rearrangements within cells as small as 20 nm, or 5,000 times smaller than the width of a human hair. These alterations are too small and subtle for human observers to detect with traditional methods.
‘The resolution of these images is powerful enough for our AI to recognise specific patterns and differences with remarkable accuracy, including changes in the way DNA is organised within cells, helping to detect alterations very soon after they occur. We believe that, one day, this kind of information may allow doctors to gain time to monitor disease, personalise treatments and improve patient outcomes,’ says ICREA research professor Pia Cosma, co-lead author of the study and a researcher at the Centre for Genomic Regulation (CRG) in Barcelona.
‘Face recognition’ at the molecular level
AINU is a convolutional neural network, a type of AI specifically designed to analyse visual data such as images. Examples include AI tools that allow users to unlock smartphones with their face or others that autonomous cars use to understand and navigate environments by recognising objects on the road.
In medicine, convolutional neural networks are used to analyse medical images such as mammograms or CT scans and identify signs of cancer that the human eye might miss. They can also help doctors detect abnormalities in MRI or X-ray images, helping to make a faster and more accurate diagnosis.
AINU detects and analyses tiny structures inside cells at the molecular level. The scientific team trained the model by feeding it nanometre-resolution images of the nuclei of many different types of cells in different states. The model learned to recognise specific patterns in cells by analysing how nuclear components are distributed and organised in three-dimensional space.
For example, cancer cells have distinctive changes in their nuclear structure compared to normal cells, such as alterations in the way their DNA is organised or the distribution of enzymes within the nucleus. After training, AINU was able to analyse new images of cell nuclei and classify them as cancerous or normal based on these features alone.
The nanometre resolution of the images allowed the AI to detect changes in the nucleus of a cell just one hour after it was infected with herpes simplex virus type 1. The model can detect the presence of the virus by finding small differences in DNA density, which occurs when a virus begins to alter the structure of the cell’s nucleus.
‘Our method can detect cells that have been infected by a virus very soon after infection begins. Normally, doctors take a while to detect an infection because they rely on visible symptoms or larger changes in the body. But with AINU, we can see small changes in the cell nucleus right away,’ says Ignacio Arganda-Carreras, co-author of the study and Ikerbasque associate researcher at the UPV/EHU and affiliated with the FBB-Instituto Biofísica and DIPC in San Sebastián/Donostia.
‘This technology can be used to see how viruses affect cells almost immediately after entering the body, which could help develop better treatments and vaccines. In hospitals and in the clinic, AINU could be used to diagnose infections from a simple blood or tissue sample, making the process faster and more accurate,’ adds Limei Zhong, co-lead author of the study and a researcher at Guangdong Provincial People’s Hospital (GDPH) in Guangzhou, China.
Laying the groundwork for clinical preparedness
The study authors caution that important limitations still need to be overcome before the technology is ready to be tested or implemented in a clinical setting. For example, STORM images can only be taken with specialised equipment normally found only in biomedical research laboratories. The installation and maintenance of the imaging systems required for AI is a major investment in both equipment and technical skills.
Another limitation is that STORM imaging analyses only a few cells at a time. For diagnostic purposes, especially in clinical settings where speed and efficiency are crucial, clinicians would need to capture many more cells in a single image in order to detect or monitor a disease.
‘There are many rapid advances in the field of STORM imaging, which means that microscopes may soon be available in smaller or less specialised laboratories and eventually even in the clinic. The accessibility and performance limitations are more manageable problems than we thought and we hope to perform preclinical experiments soon,’ says Dr Cosma.
Although clinical benefits may take years to arrive, in the short term, it is expected that, in the short term, IUTA will accelerate scientific research. The study authors found that the technology can also identify stem cells with very high accuracy. These cells can develop into any type of cell in the body and are studied for their potential to help repair or replace damaged tissues.
NIAU could make the process of detecting stem cells faster and more accurate, and would help make the resulting therapies safer and more effective. ‘Current methods for detecting high-quality stem cells are based on animal testing. However, all our AI model needs to work is a sample that is stained with specific markers that highlight key nuclear features. As well as being easier and faster, it can speed up stem cell research and, at the same time, contribute to the shift away from the use of animals in science,’ concludes Davide Carnevali, first author of the study and CRG researcher.